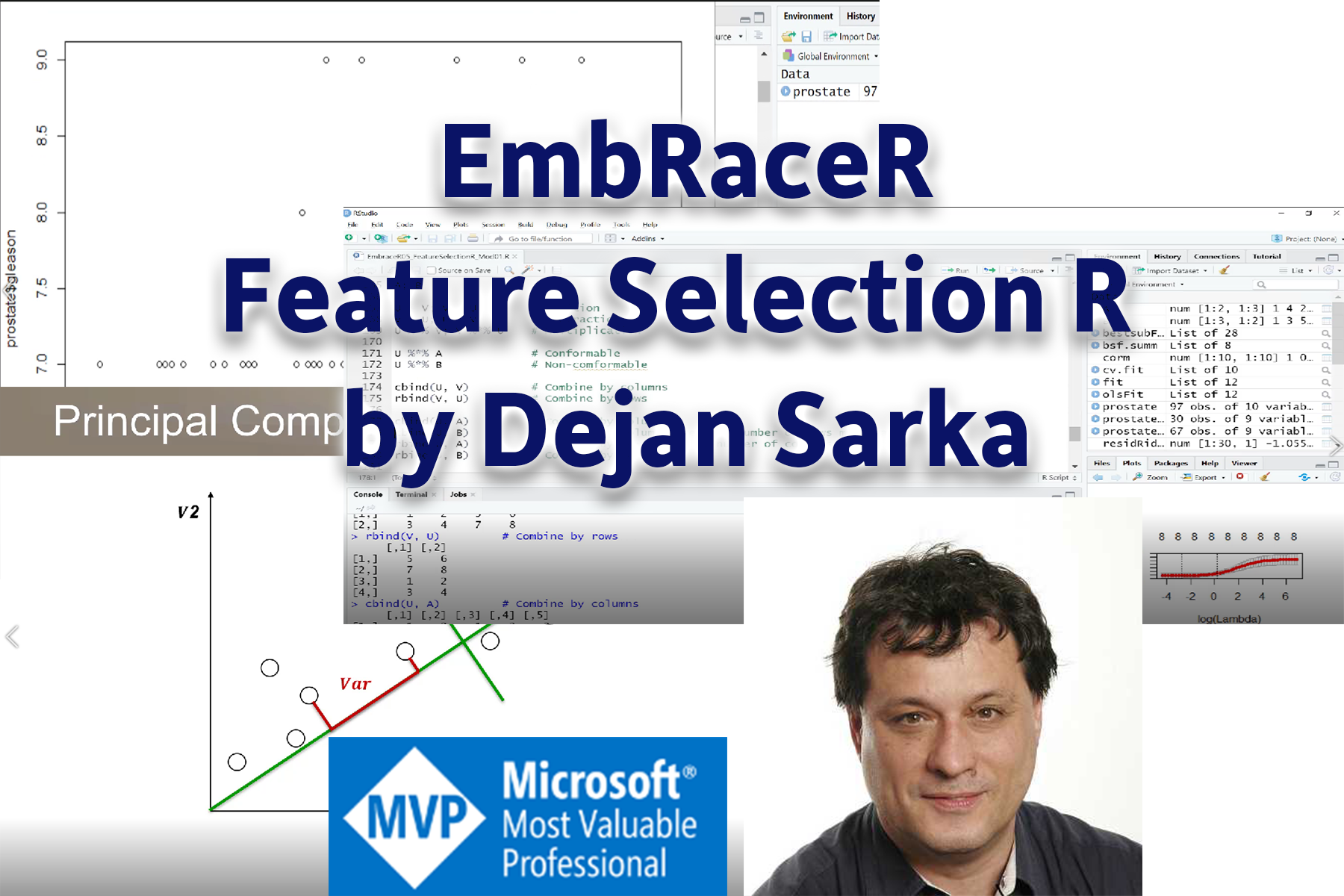
Too many variables in a model can make its own problem. This is the fifth course in the EmbRaceR course set and shows how to do feature selection, including introducing the basics of matrix calculations.
Many methods evolved for optimizing the number of variables in linear models. You will learn about forward and backward stepwise regression, best subsets regression, and then about ridge, lasso, and elastic net regularization methods. The first module also introduces basic matrix operations. This lesson is a preparation for the second module.
Principal component analysis (PCA) stems from matrix algebra and is probably the most widely used feature selection method. PCA is also useful for anomaly detection. Very similar to PCA is exploratory factor analysis (EFA), where you try to find hidden latent variables, called factors, that might be more important to use in further analysis that the basic variables, and which also give you more in-depth understanding of the problem you are analyzing.